Viewed 7685 times | words: 11346
Published on 2024-02-15 17:50:00 | words: 11346
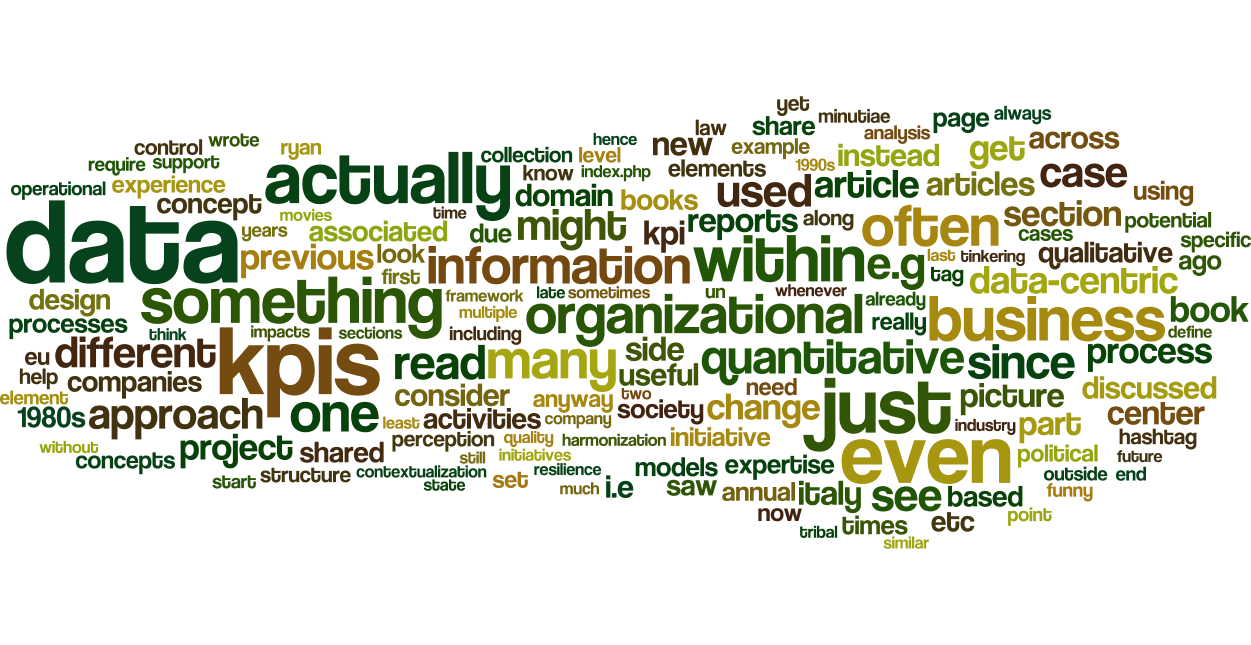
If you read the latest half dozen of articles on this website, you started with the short Moving from words to numbers: conceptual design of KPIs / progress on a data-project/data-product.
Then continued with an article that was really a mini-book (8x words as the previous one), Organizational Support 09: shuffling and pruning- an organizational PDCA proposal.
Then had two couples of articles alternating the "political" and the "business" side:
political
_ Overcoming cognitive dissonance on the path to a real EU-wide industrial policy
_ Intermezzo: structural political change in Italy in the first European election with NextGenerationEU
business
_ Change Vs Tinkering - evolving data centric project, program, portfolio frameworks
_ Designing skills-based organizations in a data-centric world: we are all investors
The connecting background concept is always the same: data-centric.
Which implies: blurring the distinction between those who produce and those who consume data, if compared even with the late XX century.
This article, leveraging in part on those previous articles, will be focused on the concepts that, from my experience since the 1980s, I think that should be used to contextualize your own KPI initiative- adapted to the needs of a XXI century where data presence, storage, processing are not an issue as they were in the late 1980s, but data quality is even more critical, as I wrote often in the past, e.g. also within two minibooks on relevant data and GDPR.
KPIs is a theme that so far discussed in at least 43 articles since 2012 (and other prior articles released online since 2003, and shared with partners since the late 1980s), and it is worth sharing another key point.
Going "data centric" does not imply turning society into a XXI century, computer- and AI-driven version of Hobbes' "Leviathan".
At the end of this article, will share a couple of book references and movies if you want to have more ideas (and develop new concepts) about the concepts discussed in the next few sections.
I do not necessarily now, in 2024, agree with all the points listed in those books, albeit both were useful while implementing management reporting and KPI initiatives, or simply having to assess the current status of initiatives and business relationships (including pre- and post-M&A, not just vendor or internal) I was asked to review or audit or help to recover/phase-out/complete.
In some other sections, will add as usual other references, including to dataset and prior publications that released online, if you were interested to read more.
This article will blend business and non-business sources, as well as reference material, as being in a data-centric society generates few practical issues that many seem to forget- more about this later.
The sections:
_ the quantitative and qualitative side of going data-centric
_ a side-effect: KPIs in a data-centric society- look at the wider picture
_ quantitative cases: UN SDGs, Eurostat, Worldbank
_ a multi-year case of designing and using quantitative and qualitative KPIs: Project RYaN
_ our own enemy: lies, damned lies, statistics
_ the oversight of KPIs: quis custodiet ipsos custodes
_ an evolving case study: assessing pre- and post-COVID impacts via financial reports
_ conclusions: some material to help look at the wider picture
The quantitative and qualitative side of going data-centric
Few days ago shared on Linkedin few posts about my business approach, generally as a repost of something that other sent me, but with my own introductory commentary (as I had been suggested by others, after seeing that my comments to their posts could have achieved better visibility that way- eventually, Linkedin added the feature).
Specifically about coaching , my business logic about coaching is that it is a tool within the framework of cultural and organizational change, with and technology.
I am not interested in decontextualized coaching, neither as a customer, nor as a provider.
Other tools that I consider critical since the 1980s (Cicero pro domo sua) are the ability to blend quantitative and qualitative elements, e.g. consider preference and existing organizational culture constraints while designing models, not just data.
For those who worked with me, probably it is not a surprise- it is the "political" element of business: if you want to implement change, you cannot rely just on data, it takes a more "systemic" Weltanschauung.
Or: you have to consider each component (including "humans-in-the-loop") with their own baggage of constraints, weaknesses, and also potential- and work accordingly to blend it all toward the intended objectives (adapting also to changes outside your control).
If in 2015 wrote a book about bringing your own personal devices at work, few years ago that evolved into you are the device.
And, as many know, now we have the infiltration of AI Chats within business, notably for lazy staff that instead of using their own company's body of practice prefer to ask a quick question to one of the various chatbots, and recycle whatever it delivers.
I have no qualms with using "smart" models, including chatbots, within a business environment- but only if their training knowledge base is known, acceptable, and consistent with your own organizational culture and, of course, compliance needs.
Because make no mistake: when we talk about "data-centric", industry is focused on sensors, devices, and also politicians seem to think about the same, plus data storage, processing, dissemination, embedding into decision-making.
In reality, as I wrote within an article that was published less than a week than the oldest in the list above, Business, policy, audiences in a data-centric society: the case of non-profit investments, you should consider the audience and revise also processes and activities that basically did not change that much since USA "robber barons" started developed the modern version of mecenatism and non-profit by building libraries, endowing universities, etc.
In the next few months, while, as I wrote recently, will increase the frequency of publications, and will explore in each article a different side of social, cultural, political, and organizational development of our data-centric society.
Obviously, while keeping an eye on regulatory (e.g. AI Act) and business evolutions (e.g. IFRS S1 and S2), but also on "feed-back cycles" that will become increasingly visible as we move more toward a "circular economy".
To repeat again: anybody who worked with me since the late 1980s (but also while in the Army) knows that I have always looked at the "systemic" side of each evolution, i.e. organizational sustainability long-term.
There is a place for tinkering, but "strategic tinkering", as I observed routinely in Italy since over a decade, is akin to trying to build a strategy by collating stop-gap measures, and then being surprised at the instability of the result.
I liked the book (albeit less some of the TV and movie adaptations) "Tinker, Tailor, Soldier, Spy" by Le Carré, but that was anyway a "closed community"- and in closed communities, one where, as said Henry Fonda in "Le Serpent" (a 1973 movie quite funny), everybody becomes "a little paranoid at the edges".
I always liked studying cultures and "closed communities", hence my focus as a kid on archeology and "dead cultures", and then on history, studies about military and related organizations, and the history of various attempts to defeat codes and rituals, e.g. a 2016 reprint of a 1994 book about Venice, Preto's "I servizi segreti di Venezia - Spionaggio e controspionaggio ai tempi della Serenissima" (read and re-read in segments: funny, if read along with De Francesco's 1939 book "The Power of the Charlatan").
Studying closed communities (even urban "tribes") actually helps to get an understanding, by comparison and difference, of the right balance between qualitative and quantitative needed within your own initiative.
As hinted above, the final section of this article will list just few business books, and many movies that could actually help develop critical thinking about KPIs and process design.
If you read so far, you understand that all the above requires data: the more data, the easier an analysis- also if you have to consider the quality of data, and what you consider "data".
Cheaper and faster storage and computing over the last decade generated a delusional state of mind, "more data equal better data".
Frankly, beside the cost of procuring and maintaing not just the data, but the associated knowledge pipeline, the more complex your system, the easier to fool it.
Our technological advantages generated "big data": which is something that e.g. would have been useful in retail in the 1990s, where instead customers told me that, due to the cost of storage and processing, they went down to details on a daily basis, rising up the aggregation level on a weekly, monthly, quarterly, yearly level.
The key element in that case was the quality and "lineage" of data: data from a checkout counter was assumed to be certified, traceable, normalized, with a known structure and content.
If you instead collect data but have no control of the lineage and quality, you end up as those multinational in the consumer products industries (fashion, electronics, etc) that had all their KPI in place, but eventually were shown to have had significant issues across their own supply chain.
Something that the OECD initiative and "OECD Guidelines for Multinational Enterprises on Responsible Business Conduct" book tried to help solve (you can download and read its 79 pages in multiple languages).
From items that will share in other sections, it is still a long journey, and for a simple reason.
As usual, if your data is self-referential, no matter through how many hoops you go, in the end, as discussed in the recent articles, you data quality is iffy at best (see Woodward's "Veil: The Secret Wars of the CIA, 1981-1987").
Now, the next section will be the longest one within this article- but, considering the "operational" purpose, it will be really a short discussion along multiple examples.
A side-effect: KPIs in a data-centric society- look at the wider picture
This section is built around examples, to show what it means to look at the wider picture.
Let's start with:
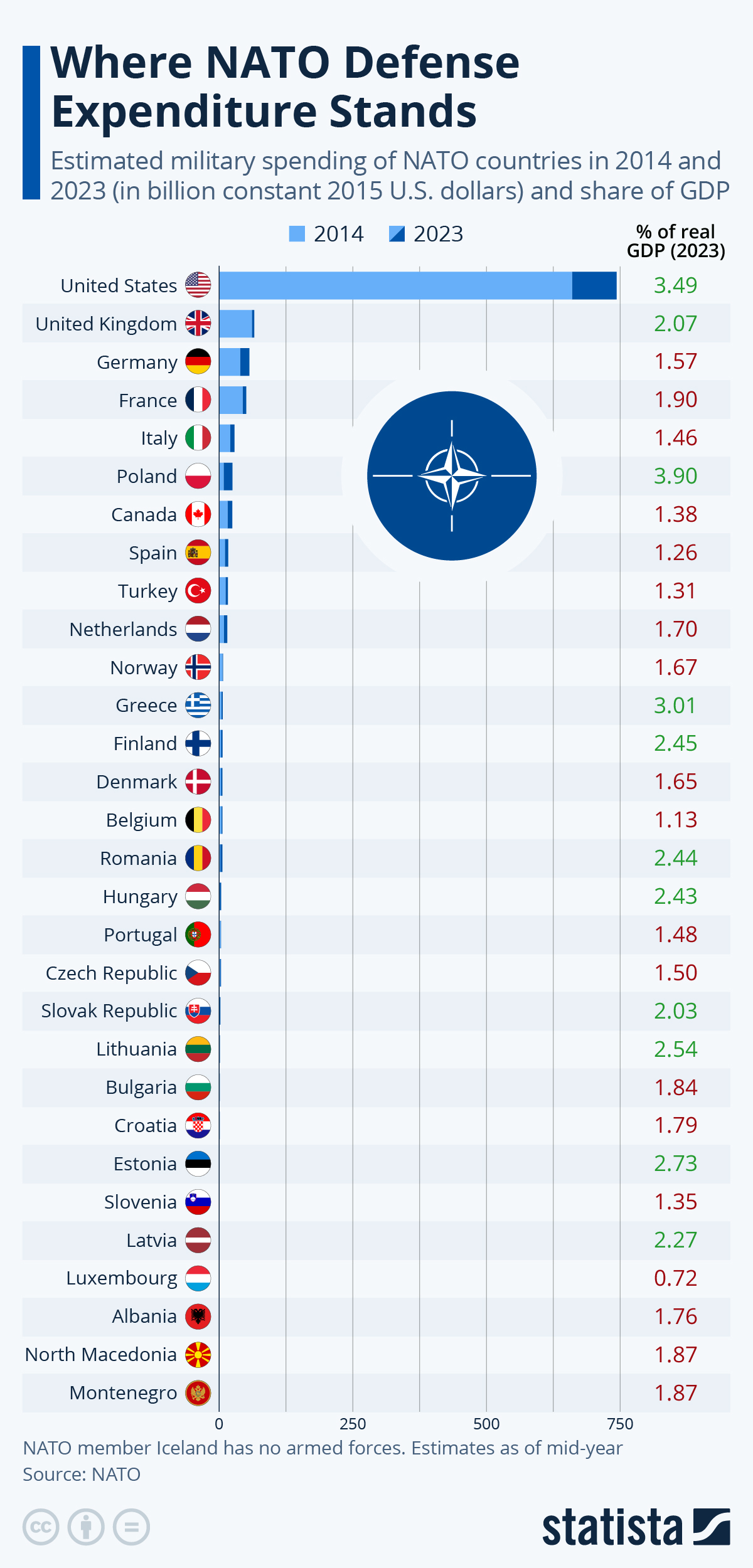
As I posted on Facebook recently:
"lies, damned lies, and statistics
the % is not the only measure that should count- the mix of tech, feet on the ground, force projection potential should matter in the latter, as shown by recent conflicts, it is true that European NATO Member States are still too dependent from the post-WWII habit to use the logistics of USA
as a Bulgarian acquaintance said to me in Brussels over a decade ago: when he was in the Army, he was told exactly what we were told in Italy few decades before- that we should resist few minutes to buffer until our "Big Brother" (USSR for them, USA for us) would start arriving
discussing about the % of GDP assigned to armed forces it is akin to the discussion about "Airbus receives subsidies"
well, military high-tech research and procurement is a form of industry subsidy, and forcing allies to allocate part of their spending to systems that support USA companies is a way to externalize costs
reminding a bit Athens' Delian league https://en.wikipedia.org/wiki/Delian_League
and increasing the tech content increases the % having just one measure to represent a complex set of factors is somewhat misleading and open to interpretations "
Let's now shift from the obvious "systemic" (as there is a long, long supply chain before you have something useful by armed forces), to something that sometimes we consider less so, but it is equally subject to "cocooning" of communication to ignore what happens outside our own organizational boundaries that makes our own activities possible- ditto for customers, who buy brands and can have complete ignorance of the associated "lifecycle" of the garment they purchase and wear.
I referred in the previous section to the OECD Guidelines.
And I actually posted something earlier this week on Linkedin:
" the #OECD #Guidelines for #Multinational Companies, that used e.g. also a couple of decades ago when asked by a customer to design a SOX-inspired oversight organizational structure and associated processes, should be a starting point for harmonization
But in #ESG times transparency across the hashtag#supply hashtag#chain should be part of any #sustainability initiative, as taught by #COVID reshoring or nearshoring will not always be feasible but, as there can be quality control on suppliers , terms and conditions of supply could embed also those elements related to the #lifecycle of supply - including the "s" of ESG "
Shifting to one of the industries where I worked more often, automotive, data-centric is taking on a new dimension, even without adding in the picture autonomous vehicles.
Modern cars and commercial vehicles are really computing facilities on wheels, and both on a regulatory and technical perspective it is becoming feasiblle to have those data leave vehicles and interact with the environment.
This article (in German) references Cloud and automotive, but actually covers also the "local" level of potential interactions ("Edge"), and gives an outline of what, transposed, can be applied to other industries.
If you read all the examples and links in this section, you saw how, often, what could seem a "local" element, within a data-centric society, which enables a continuous interaction with the environment, assumes a different dimension.
In our data-centric, UN SDG, and ESG times, a PEST should be an element within any business case.
You can search on Wikipedia about both ESG and PEST, but conceptually the selection in 2015 of the UN SDG was a recognition of the need to consider both quantitative and qualitative elements.
Personally, since the early 1990s, whenever I was asked to work on change, or consider elements for a new system or contract or business case, always "embedded" at least what was directly affecting (and could therefore be directly monitored) the customer.
When I proposed in late 1990s to introduce such "contextual-level" elements, to start using in banking in Italy a branch-level risk monitoring tool complementary to the Italian Centrale dei Rischi, I went as far as I thought feasible.
So, "clustering" along multiple dimensions, such as industry and location, as e.g. I assumed that the construction industry in Emilia-Romagna (the Bologna region) could have a different profile from the cheese industry in the same region (where some assembly line workers told me that they got as much in "official" salary as they got in "fuoribusta", i.e. cash- needed to retain talent in an industry that was accelerating), and even the same industry in different regions.
The issue was who was going to collect and certify data, as weren't along those available, and therefore I do not know if it was eventually done (albeit, many years later, the Italian State actually did something similar, to create "parameters" to benchmark the reliability taxwise of companies- with some market distortion results).
I think that a PEST (or "contextual") approach should actually be embedded within any evaluation or performance measurement approach, and should also potentially be extended to, if not a continuous, a "time-framed" approach.
The idea is that continuously updating KPIs, also if technically feasible, has to be aligned to the ability to process the information that KPI provide.
If your have instantaneous update and need to discuss information with others, unless there is a single source of truth that is acknowledged by all with the same point of reference, the risk is that different parties will join a discussion each using the same source but, due to differences in processing times, with a slightly different picture.
It could seem funny, but, trust me- I saw plenty of discussions and diverging opinions deriving from the same source of truth, but "sampled" in a different way at different times: as somebody said, if you torture data long enough, will tell you anything you want.
My first project with a large KPI-based system was in 1988, and involved a group of companies that had to report monthly.
The larger the number of contributors, the more the design not just of the KPIs and reporting, but also the process and data pipeline become critical.
It is again a case of "contextualization": as I wrote in many previous articles, a system designed assuming that all those contributing have the same organizational structure that you have might generate significant overhead and re-processing along the line, re-processing that could turn into plain "data morphing out of shape".
Another contextualization point never to forget is the motivation of those providing the data: harmonization at the top is fine, but if those providing data have a vested interest in what the data present or their perception at the top, some "fixing" along the road should be considered a risk.
Quantitative cases: UN SDGs, Eurostat, Worldbank
This section could be boring- so, instead I will share just a list of datasets where you can explore the concepts, and few concepts.
The concepts first: as discussed while closing the previous section, in large enough organizations it is normal to have a need to orchestrate priorities based on how operational units are performing.
Monitoring performance is just one of the elements: you have to collect also the meaning from the operational side, and have access to information underlying what is being shared after harmonization.
Again: those who worked with me know that, probably coming from my political activities number crunching before starting to work, or my listening as a young kid about accounting for my parents' small company, I am used to see numbers as contributing part of a whole.
Hence, I found annoying whenever data are obviously "padded" without any corroborating information or traceability to the lineage, i.e. understanding where the information comes from.
Yes, when asked, as it happened once in a while in business, did take existing unexplained information and devised an algorithm to "project into the future" (e.g. building a new budget based upon an existing one plus organizational changes), but then I like to attach to each made-up figure the source, the rationale, and the positive/negative choices taken.
Reason? To create a baseline that others could evolve on, or refute, or restructure, you need to explain your numbers.
Even more when numbers are a discrete representation of subjective information (e.g. ranking your impressions on quality instead of reporting actual measurements on number of failed parts, etc).
As discussed in the previous section, a further element is the set of organizational (and human) capabilities available, and factor them within your definitions.
Example: if computing your KPIs requires knowledge of accounting and finance, linear programming, etc to compute a single value that has then to be transmitted and consolidated along with the other values from other units, but most of your units have just operational resources and rely on a central structure or outsourced activities, then adding this overhead could either imply additional costs, or having somebody add up apples and pears.
The UN SDGs have a quantitative element and a qualitative approach (and viceversa- depends on your political hue).
Personally, I think that both the qualitative and quantitative elements require an integrated approach- and shared in previous articles the issues associated with trying to achieve a EU-wide convergence on UN SDGs.
I shared in the past a dataset (UN SDG - EU 27 sample datamart (subset for 7 KPIs)) where selected a sample of 7 KPI EU-wide, and actually could be interesting (and might do it) to see the evolution of those KPIs before and after the completion in 2026 of the activities related to NextGenerationEU, its associated Recovery and Resilience Facility, and other further initiatives spawned since 2020.
Actually, in September 2022 released a further dataset with the initial assessment (rating) of the plans provided by each EU Member State.
Again, the idea was to benchmark EU Member State(s) vs. a shared, agreed framework.
As outlined within the official presentation page:
" The Facility entered into force on 19 February 2021. It finances reforms and investments in EU Member States made from the start of the pandemic in February 2020 until 31 December 2026. Countries can receive financing up to a previously agreed maximum amount.
To benefit from support under the Facility, EU governments have submitted national recovery and resilience plans, outlining the reforms and investments they will implement by end-2026, with clear milestones and targets. The plans had to allocate at least 37% of their budget to green measures and 20% to digital measures.
The Recovery and Resilience Facility is performance based. This means that the Commission only pays out the amounts to each country when they have achieved the agreed milestones and targets towards completing the reforms and investments included in their plan. "
I will let you read the material provided at the links associated with the dataset, and decide for yourself if the issue was the design or the method or both, but we still have a couple of years to go.
There are further "benchmarking" datasets that shared on my kaggle profile, in some cases also with a companion webapp to navigate the information, and presentation notebooks.
The title of this section lists three of the sources I used for those datasets, but used also other national sources, e.g. a Ministry for data about State libraries from 1994 to 2018.
If you read the description of each one of those datasets, and have a look at the data, you will see that are all about comparing, benchmarking, harmonization.
And you will see that it is not as easy as it seems...
... even if you stay just on the quantitative side.
Now, what happens when you need a blend of both quantitative and qualitative elements?
Will share a real example with documentation that is close and related to a couple of early 1980s nuclear war scares.
It starts with something simple, KPIs, but I will share considerations also about the process.
On the latter, more a couple of sections down the road.
A multi-year case of designing and using quantitative and qualitative KPIs: Project RYaN
You read above and in previous articles how I consider that, in our times, it is even more critical that we get used to the consider "the whole picture".
That does not imply "everything under the sun"- but what you can assume to be relevant on at least three counts:
_ it is under your control and impacts your initiative
_ it is under your control and impacts third parties
_ it is not under your control, but impacts your initiative
_ it is not under your control, but could generate via third parties impacts on your initiative (including their perception of your initiative).
Admittedly, the last two require monitoring and "tea leaves" approaches, while having also some contingency planning and potential remedy measures.
The first one, unfortunately, is often the only element that is really taken care of.
The second element assumes shifting mindset, and considering perception and motivation of those third parties- which implies further... monitoring, contingency planning, and adaptive management.
Sometimes, it is not what you mean or your intent, but its perception that matters.
A much discussed case that was until few years ago a "tribal perception" matter is something that happened in 1983, when a set of massive yet routine training exercises (was done e.g. also in 1987) generated some reaction based on "what if".
Or: "what if" the readiness and preparation exercises are really a cloaked mobilization?
Sounds preposterous, but actually in a paranoid mindset makes sense.
The point is: having a clear perception of reality.
As I shared few days ago on social media, the introductory part of the book " Able Archer 83: The Secret History of the NATO Exercise That Almost Triggered Nuclear War" is an interesting reading- supported by documents that have been made public over the last decade.
So, if you are interest in reading not just the storytelling of what happened, but actually (the part that has been released) of an internal ex-post assessment, have a look at that book.
This introduction is not to scare you (we live in similar times now, with a couple of live conflicts ongoing at the border of Europe, conflicts that might expand), but just to contextualize.
I will spare you movies from the early 1980s, from "The Day After", to "Wargames", to "Threads", movies that represent the mood of the times- watched now (as I did after re-reading the book and reading the Project RYaN material), are mildly depressing.
You can read an outline on Wilsoncenter.org, where you can find also all the documentation that I will reference in this section.
I will focus on Project RYaN itself, i.e. a KPI collection that was to feed a decision support model.
What was the project?
From that website:
" Project RYaN was a KGB lead program to anticipate a "nuclear missile attack" (Raketno-Yadernoe Napadenie) on the Soviet Union. Beginning in 1981, the KGB operated a worldwide intelligence network to monitor indicators and assess the likelihood of a surprise nuclear missile attack launched by NATO. The collection contains translations of documents released by the Federal Commissioner for the Stasi Records (BStU) which show KGB/Stasi collaboration and casts an unprecedented light on the operational details, structure, and scope of the RYaN project. "
This picture represents the indicators identified and discussed in a document of 1984-11-26:
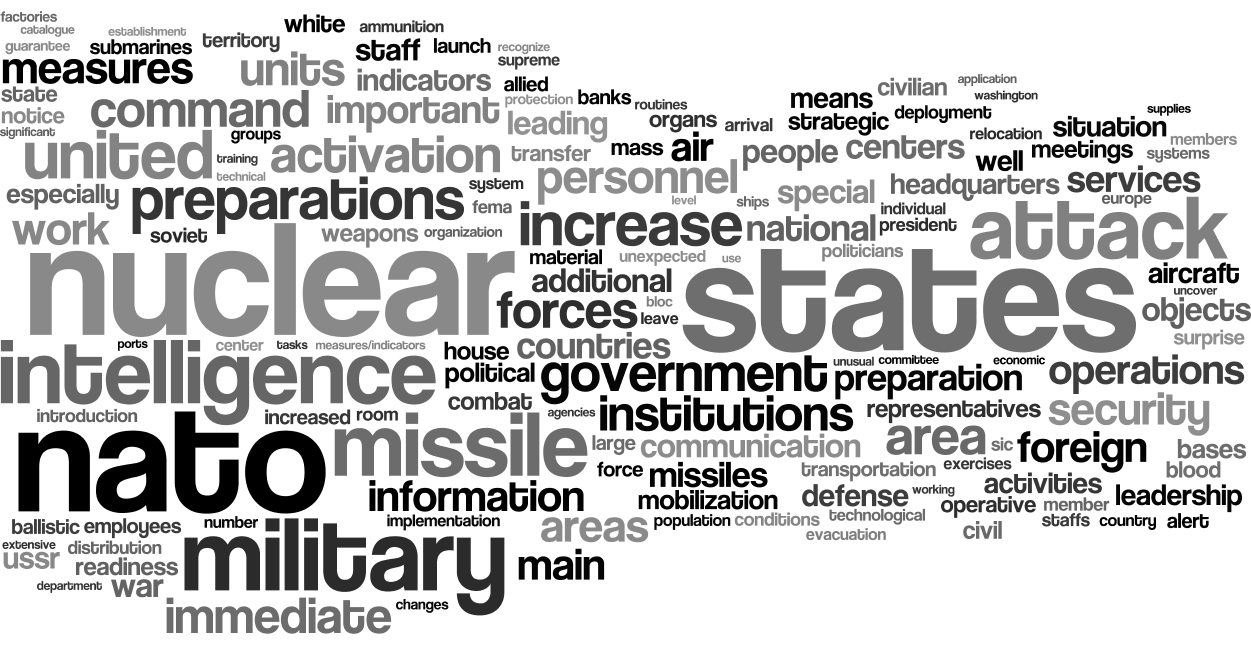
This picture represents a development report of 1986-05-06:
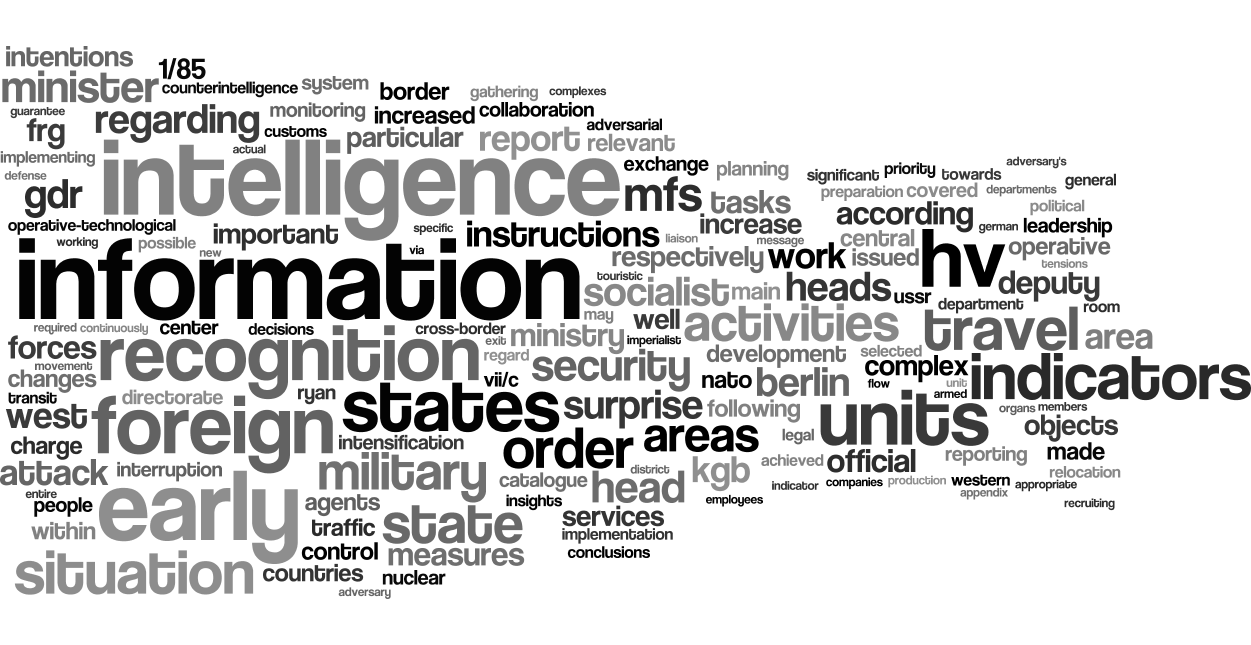
It was then followed by monthly reports- the Wilson Center website collects a few spanning from August 1986 until April 1989, that I conveniently summarized for you in this small animation:
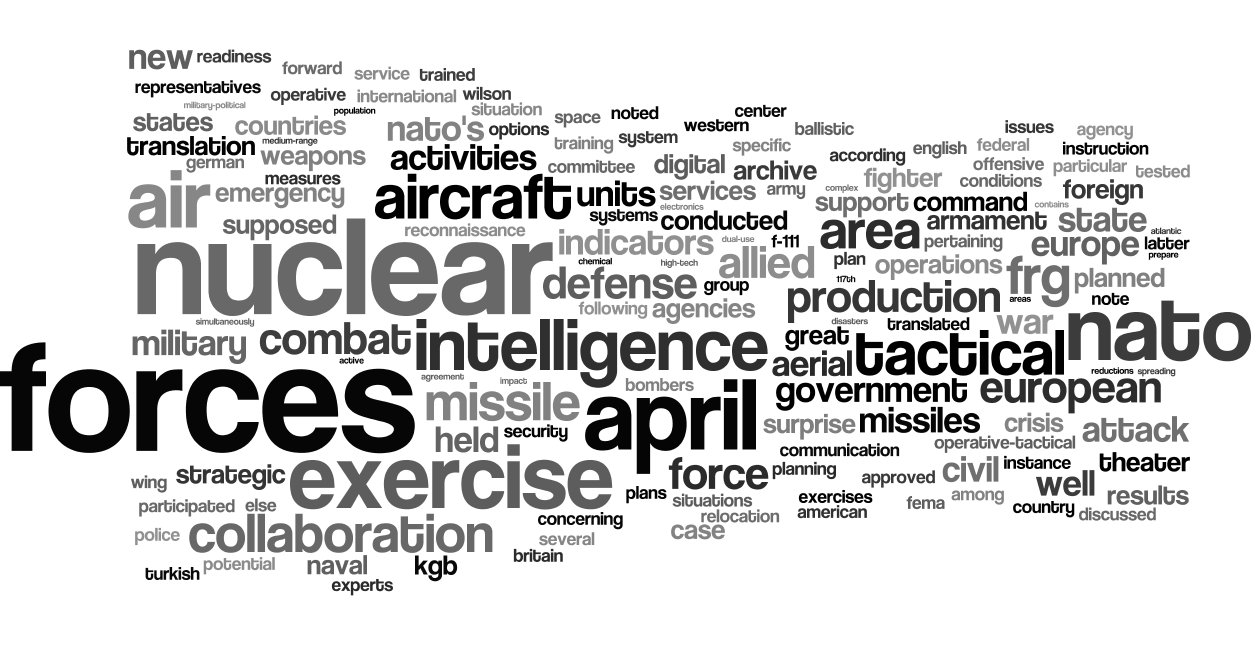
A tag cloud is purely quantitative (shows how often a word appears in a text).
Within the context of status reports, sometimes make sense (as what I derived from quickly reading those reports was roughly aligned with what each tag cloud represents), but in other cases delivers the typical bias of any supposedly quantitative analysis.
Unless you are trying to sell something and at the same time evangelize the market about your new wordsmith-derived "verbal jingle", in a report what it is about does not appear more often- you should instead build a tag cloud based on associations, i.e. the context where each word appears.
When you shift from purely quantitative to a blend of qualitative and quantitative, direct understanding of non-verbal and contextual information becomes even more relevant: i.e. you need to associate numbers and other information, and how both are connected.
Why "even more relevant"? Because also pure numeric information might be associated with a specific context.
Tag clouds are useful yet potentially misleading tools, and before discussing the oversight of KPIs, a discussion based on example from other domains.
Our own enemy: lies, damned lies, statistics
In Europe the ECB inherited the German obsession with inflation rate linked to the aftermath of two World Wars in the XX century- but inflation rate might derive from internal or external reasons.
If you, as the EU Member States do, import most of your energy, and you have an industrial economy with significant use of energy, part of your inflation is outside your control.
Unless you cut down energy consumption which, in the short-term (as in the long-term hopefully, as the EU is doing, you shift sources), implies that you cut down production and hence turnover, there is a part of that "simple" figure that is really outside your control.
There is an attitude that increasingly saw developing within the EU that I nicknamed the "Gosplan attitude"- the idea that you get a bunch of experts, put them all together, and they will have universal knowledge to dictate details to everybody else.
It might start as a team effort to collect and collate, but eventually that team effort results in detachment from reality, as it is a nuisance to talk with those who do not share your own lingo and procedural savoir faire.
Actually, gradually that team effort expands, and detachment increases, while "productivity" of that team of experts expands.
The result is what we saw recently in changes on the agricultural policy: the French approach to protests related to agriculture (blocades by tractors) won a (temporary?) reprieve on the mandate to let 4% of land to rest on a rotating basis and other components of recent reforms.
I know that a Japanese friend told me that "ringisho" is slow.
But if, as the EU does, you advocate for subsidiarity (to have decisions made at the lowest point possible), then you cannot continue, in the XXI century, to use a technocratic variant of the "Monnet" jumping forward, which was initially used at least by elected politicians (so they had a mandate) to avoid continuous re-negotiation with their internal opposition even after having won elections.
I did not like it that much back then, even less so when it is de facto applied by unelected officials that then push it through political approval, as it frankly has been increasingly so.
Anyway, we will see in few months if politics will take again the full initiative at the EU level.
Now, when I wrote "politics", I meant "elected politicians with a mandate".
This apparent digression was actually to discuss on something closer to the aim of this section: what happens when, instead of having both data and "sentiment" filter up from the ground to the decision-making level, you transfer just data and only data that are perceived as acceptable.
You can read the documents on the Wilson Center website, but I found the same issue since the 1980s in many KPI/performance initiatives and decision-support managerial reporting.
Even if you count widgets (or, in the case of Project RYaN, even the increase in blood provisioning or traffic between leaders), if you do not see the whole pipeline (e.g. raw materials vs consumption rate, losses from mismanagement in production or even transportation, what is already finished yet in store to be sold, what is under rework), you risk getting the picture that confirms your views, not to derive a view from pictures.
When you shift to non-quantitative data delivered directly or even converted into quantitative measures, the issue becomes decoupling collection from analysis and contextualization- at the top (where, as in this case, analysis reportedly was done), but also the bottom (where was reported that explicitly had to avoid doing any analysis).
Personally, what I observed since the 1980s is that, where data are collected, you should have both, but clearly separated, as collection is anyway biased, and trying to "objectify the subjective" often ends up on second-guessing what could be acceptable, hiding or inflating, again subsidiarity is the key.
There might actually be multiple levels of "collectors", some basically collecting and bean counting, and others doing a contextual analysis "in situ" before aggregating or even just transmitting.
Data collectors receive instructions on what to collect, but then have to convert that into what is feasible and available, and, unless they are involved within the design, often will do as in Apollo 13, fit a square peg in a round hold- or viceversa.
For that NASA mission was an engineering challenge but helped to overcome an obstacle.
In business (but also other environments), it is often not even known that such "squaring the circle" is carried out, and could turn into a collection, making meaningless concepts as "business continuity", or even "traceability".
If data or commentary are tweaked to align with expectations, eventually build their own "tunnel vision".
If you are on social media, their embedded "recommendation system" is giving you a taste of what it means.
I have fun sometimes by trying to fool Facebook or other social media in redirecting what they show in my stream, and sometimes is really funny.
Trouble is: if you enter a "conspiracy theory" stream, then most of what you receive is aligned with what you saw.
And the more you click or read some of those items, the deeper the tunnel becomes.
Hence, in this specific case, everything will turn into a conspiracy.
With KPIs, it could end up in generating a self-fulfilling prophecy: you get distorted information from the ground that confirms your theory, pass over the message, and you keep getting more distorted information.
It is used in some organizations for "plausible deniability"- by design or by change.
If you do not know because information is "filtered" before reaching you, and, once received, you ask for more of the same, you will get more of the same.
In the last section of this article will share books and movies that could deliver something that could easily require 10-20k words.
I do not expect that you will watch all of them, but if you do, you will get an overview of different issues on collecting information, reporting information, and making choices based on partial, misleading information, or simply incomplete information.
As stated above in the longest section, while discussing the percentage concept for NATO national expenditure, trying to "harmonize" could flatten differences but, if that harmonization is top-down, as often happens, it becomes experts-driven.
Harmonization experts-driven often is a kind of horse designed by a committee that has to achieve a consensus: a camel.
Discussed already in previous articles EU's desire expressed also as part of NextGenerationEU and associated components to "converge" on a specific profile on UN SDGs alignment across all Member States.
The Recovery and Resilience Facility was an opportunity to rebalance, but its implementation was again top-down.
As discussed in previous articles, more than once the "sentiment" was as if national governments were feeding within their plan what they could not get through their Parliaments, so that could claim those "objectives set by Brussels".
In the case of Project RYaN, and other KPI or PEST initiatives following the same approach, while central analysts might focus on the wider picture, by microanalysis at the centre would risk projecting their perception on what the data really contain, further distoring the picture.
As you can see from the tag cloud across time animation, perception evolved and it is difficult to say if, in that case, data was leading or following perception.
Hence, oversight of KPIs across their lifecycle is something that needs to have at least two layers, as any cultural change initiative (as usual, with or without technology): a "helping you continuously to improve and share lessons learned" side, and a "compliance" side.
The oversight of KPIs: quis custodiet ipsos custodes
I know- I used that phrase quite often.
Within the scope of this article, it actually takes on a slightly different, and more structural, meaning.
If you read my previous articles, you know what I wrote about watchdogs.
When defining KPIs, a clear concept that is often lost between the minutiae of measurement and performance is something that is straightforward and does not require specific domain expertise: due process.
The idea is simple, and is the same that adopted elsewhere when working for customers: KPIs are elements within a "social construct", actually a "social contract".
When you define such a structure, you do not just collect a mix of details, you have an overall, uniting logic that defines also what is in, and what is out.
Also, you need to have and manage a lifecycle for each KPI: some organizations instead seem to fall in love with their KPIs.
While the actual convergence of operational data into an harmonized, shared KPIs might require expertise, the process followed should have some "milestones" that are followed across the board by everybody in every domain- e.g. data considered should not appear out of thin air, changes should be traced and justified, etc.
In Italy, I already criticized decades ago our approach that I saw whenever I had to help a customer to define requirements for compliance based upon a new law: we deep dive into minutiae, and then add "connective tissue" to hold our new Frankenlaw together.
Then, we start tinkering as tribal pressure mounts, whenever there is a chance that the balance of tribal power can be different from that one available when the law was designed, or even voted.
Specifically, we have too many specialists who have their own domain expertise and often "project" that, and the minutiae associated also with their tribal demands.
Having a domain expertise helps, as you can grasp if a due process was followed or not, also within other domains outside your field of expertise.
If you are "structured" in your own domain, you probably have some patterns.
When you deal with some who are outside your own domain of expertise, after few years you get used to differentiate between those who have different yet defined patterns, and those who are simply chaotic or pretending.
For example, whenever I met entrepreneurs or startuppers, or even when I was asked to help recruiting either staff for a mission or a new partner, I might not be an expert on the specific domain, but was looking for something that showed patterns acquired through experience.
I remember meeting in Brussels a recruiter who said that he had previously hired somebody who, after being sent to the customer, was proved to be just a talker who had read some books.
Which is, actually, the feeling of many who read my CV- and often I wonder what would say if they were to read my real, full-length CV.
Brussels was funny- more than once received a scheduled job interview where, at the interview itself, was disclosed that they looked for somebody with a different skillset.
Even funnier when I had to quarrel with recruiters who actually asked to "tweak" my CV so that would fit their job description- by adding fake or misleading information.
In Italy, as shared in previous articles, the issue is often the other way around: apparently, many have budget for something, but then would like something they did not have budget for.
No wonder then that companies complain of having many positions unfilled.
It is curious how this environment, when looking for people to elect to the Parliament, apparently continuously asks for "competent" people even when competence in a specific domain, if becomes your tunnel vision, could actually be detrimental.
To say nothing about those who have no competence or even no experience whatsoever, and somehow get through all the filters, true to the Peter Principle.
Put them elsewhere, would minimize the damage.
Put them in an elective office, and they can maximize the damage they generate on society while massaging their own inflated ego.
Anyway, also some without competence or experience might actually "learn the trade", and gradually become asset to the State (and its citizens).
The key concept here is: when elected into Parliament, where one day you vote on A and another on B, you should anyway become a generalist most often than not.
Two examples: our watchdogs and how the law on torture has been so far applied in Italy.
Our watchdogs often seem to be catching the news wave by following news headlines, not with a structural design: a collection of high-visibility minutiae that makes our complicated society not just complex but even chaotic, as I discussed in previous articles with reference to the legislative and regulatory process.
If you catch the news wave, you issue fines etc, but I would frankly prefer something similar to the approach of GDPR: you have a framework, and the minutiae derive from that.
If you violate the processes set within that framework, computing penalties about the minutiae follows, but violating the processes is already enough.
Moreover, processes are shared, transparent, not a whimsical ex-post Draconian implementation of judicial will.
In Italy, traditionally until few decades ago we had an approach that could be summarized as "you can do whatever is allowed"- hence, our staggering number of laws and regulations.
In more recent years, we blended that with a kind of ex-post regulation and linkup to specific cases, something that reminds more the Anglo-American approach.
In the end, we got a kind of hybrid, and instead of the "libra" that you see in tribunals, we ended up with a pendulum that swings à la Poe.
Ditto for our belated law on torture, that we were supposed to have in Italy as signatories of international treaties long ago, but really had only after individuals, following the Genoa G7, took the time (over a decade) and money (I remember 70k EUR) to get through all the judicial levels in Italy, and up to Strasbourg.
I wrote "invoked", but I should say "evoked"- as an ectoplasm: so far, its application has been closer to the use of a ouja board than application of law.
Curiously, reminded me of the USA discussion about waterboarding etc: I do not care how many times and for how many minutes, or which level the pulse and pressure of the individual reached, to define if it is torture.
A due process approach would simply say:
_ why did you have them in custody?
_ what were rights and duties while in custody?
_ were those processes followed or not?
I do not remember any legislation in Western countries that stipulates how many times you can beat a prisoner, or how many minutes you can waterboard said prisoner.
As in modern countries you do not legislate about "acceptable torture".
The idea is that you are a prisoner, hence under surveillance but also under protection, and that your "guardians" are called such, not "temporary punishers".
Back to the legislators example.
If you focus on minutiae, either for your own domain expertise or tribal demands, the law that "emerges" from that collection (more, collation) needs some stitching, to blend all the elements.
Moreover, if I am an expert in A, and my tribal demands require B C D, I end up relying on "experts" that might have vested interests of their own.
So, you end up with some laws and decrees where, as an intellectual exercise, already decades ago in my spare time searched for "tribal earmarks".
on Italian newspapers, notably since the 1990s, when the Second Republic started, once in a while the new laws, which were skeletons to be filled then by bureaucrats, were described as subject to the intervention of "la manina", i.e. the hand of somebody who added details that actually re-routed the purpose.
Therefore, since 2012 I saw often ex-post tinkering: and I will let you think how chaotic this makes the "rule of law".
I already had my first experience with "retroactive laws" when I filed my first tax return in the mid-1980s, and frankly was curious to see the movie from Costas Gravas "Special Section" about an episode that did not know, the case of a retroactive law during Vichy and then what ensued.
Also in business, if you do not have an overall concept, and rely just on individual experts (often outside your structure), you end up following what I call the Frankenlaw approach: a bit from Germany, a bit from UK, a bit from USA, a bit from France, and here comes the new Italian law.
That the "ensemble" is not an orchestra but a cacophony, that of course requires stitching before the routine tinkering even starts, seems to elude many.
As I said often in business when working on change or revising processes and systems: adapt before you adopt.
Just because something is a best practice elsewhere, where a different "context" (organizational culture, legal framework, economic conditions, etc) is aligned to that (and viceversa) does not imply that such a "best practice" will work for you.
Actually, adopting a best practice that does not fit your organizational capabilities might be detrimental, as I said when a smaller company purchased a larger one delivering similar services, and one of the first steps was to hire as a director in charge of accounting somebody coming from a huge multinational.
I said: give "responsible of" as title, not "director of", as otherwise, as soon as he is hired, will start building his own small empire to replicate processes and structures he is used to.
And, pronto, I was a prophet: the newly hired "director of" immediately needed new staff, and soon the common complaint was that they had a pile of additional paperwork to do just to deliver the same results and without any significant increase in staffing on pre-existing activities.
Therefore, also on KPIs, I saw that often what makes sense is something different:
_ define the concept and purposes
_ define the draft due process
_ negotiate each KPI with domain experts, but considering also if their motivation contradicts the concept and purposes
_ finalize the due process
_ have a structure that takes care of KPIs, but contains two substructures, one focused on supporting implementation, one on monitoring.
The last row deserves an explanation: in political activities, but also in the Army as "furiere" (anything from scheduling daily services, filtering request for R&R, supporting those taking care of field exercises, etc, plus my other volunteer roles that you can find online on my CV page), well before my official business activities started in 1986, saw that there could be a conflict of interest in having to cover both roles.
Avoidable only if you are willing and allowed by your organization to "compartmentalize" your own activities.
Because that advisory role is important to get feed-back and identify potential tuning needs, while the compliance role, if manage with multiple levels as any formal compliance, could actually also help structures to evolve.
For example, when delivering methodologies and associated training and coaching, I was sometimes in the unusual position where I had to refuse to the customer information that, under the first part of the role, I had been given "in camera caritatis" by their employees.
I was lucky to have clearly defined the boundaries of my mandate with the top level of the company in each case, and when explained the rationale, was authorized.
The idea is that the "advisor" side helps to pre-empt that the monitoring side will find failure of compliance, and collects signals from the frontline.
In some organizations, instead KPIs are parachuted on those who have to provide them, and the game is really a kind of "gotcha".
In those cases, many simply adapt to survive- and I saw plenty of "creativity" in numbers, creativity that could be understood only if you had domain expertise.
Anyway, when you shifted to the due process approach, basically stating that their domain expertise was their own, the KPI had been negotiated, but they had to follow a due process and provide lineage and traceability, even a non-expert could identify "decision-making troublespots", i.e. where some logic steps had been replaced by "gut feeling"- often, based on vested interest.
Example: say that you want to get from your sales managers their forecast for the next year, and then monitor them on that, and bonuses will be based on the usual On Target Earning (i.e. you get it if you do the target).
If you lack the expertise to assess the meaningfulness of those figures, you will probably have to look at a bit of history down the road, and see how many as if by magic reach always their OTE but, if you set a "cap" (a limit on how high the bonus can go), apparently they never go that much further.
A due process analysis might actually see how many cases of negotiations are dragged into the beginning of the next year, but older hands with long term relationships with customers might actually "spread" that across time, while younger ones might reach their target as soon as possible, and then apparently for months chasing their own tail.
Anyway, back to the legislative example, when you have non-experts focusing on minutiae, or even experts doing it outside their own domain of expertise, what you get, beside the obvious "earmarks galore" (yes, a reference to the 1949 movie "Whisky Galore" about a beached ship filled with whisky), is an additional risk linked to tinkering by non-experts, i.e. their inability to understand the whole picture.
Will discuss this in a future article, but for now just keep in mind this point.
Now, as part of my data-centric projects, in 2022 started looking at companies listed on the Italian stock exchange Borsa Italiana.
Of course, a project focused on deriving KPIs from data.
An evolving case study: assessing pre- and post-COVID impacts in Italy via financial reports
This is a short section to discuss how the approaches presented in previous sections were applied to this specific project.
For those who have not yet read about it: simply, in 2022 decided to test some of my new and refreshed skills, including on building and using models, by doing a quantitative study.
To summarize what happened since fall 2022 (you can follow evollutions here):
_ looked at the presentation pages of all the companies listed on the Borsa Italiana
_ defined some criteria (basically: transparency, as shown by public and free availability of information)
_ retrieved annual reports for 2019 and 2021
_ selected a subset of companies, those with a stock identification number starting with IT (technically called "ISIN") and annual reports also in English
_ studied reports to identify potential KPIs to fit my purpose, i.e. comparing 2019 with 2021 to see COVID impacts
Then added further selections and restrictions.
The KPI I identified? Will share in a future article, but in this one instead would like to share what I obtained by following the approach discussed above.
A short list:
_ as annual reports figures are to be contextualized with what the annual report describes as choices, selected an external, harmonizing source
_ considered from Yahoo finance, for the companies within the selection, the 2021 data, and compared what was within Annual Report with Yahoo computations
_ identified the patterns adopted by each company, as probably a similar pattern will be also in annual reports for 2019
The interesting part?
As an example: If I had used just the annual reports data without looking at the associated descriptive notes, in some cases would have considered e.g. as debt toward suppliers what actually is debt toward employees (in Italy, each month an amount is set aside; historically, many companies use it as an additional source of cash to finance themselves).
Further examples? Well, let's just say that whenever I found a discrepancy between Yahoo finance and annual reports, if it was possible to reconcile, the difference was always due to similar expansion/contractions.
My favorite? Seeing marked as account receivable what had not yet been billed to customers.
Reconciling those 2021 annual reports with Yahoo finance for the same year, and referencing to the explanatory notes, helped to highlight practices adopted by each company.
The concept is simple: I followed the process described in the previous sections to define the KPIs I would like to use and the companies involved.
This helped to see that just because a company labels something as "Receivables" or "Payables", does not imply that an harmonization party (Yahoo) that has to present the same logic across the board would recognize them as such.
Actually, some of the different concepts I saw across companies were something I already saw since the 1980s in annual reports.
Meaning: things did not evolve so much, since then.
Still, between Yahoo finance used to highlight discrepancies and explanatory notes to be used to reconcile those differences, ended up with a "level playing field" to avoid aggregating apples and pears.
Conclusions: some material to help look at the wider picture
Writing this article (minibook on KPIs) was for me an interesting travel through memory, updated with some more recent information.
If you read so far, I hope that was as enjoyable to read as it was to write.
The key concept is: KPIs have to be contextualized- both as content and as structure, and obviously also as processes and organizational structures.
If you have a small company, as I did when I had my own Ltd, anyway having a model with some KPIs allowed to know when to turn down offers that would have made not possible to develop activities whose results ended up being pivotal in other activities.
As promised, would like to suggest few books and movies.
Let's start with contextualization:
_ 1971 De Bono's "lateral thinking for management"
_ 1968 Whitfield's and Roddenberry's "The Making of Star Trek"
Both books, in their own way, are about contextualization- and useful enough to have been reprinted at least until the early 1990s (when I my copies of both were printed).
Also if you are not a "Trekkie", "the Making of Star Trek" is useful as it was designed doing what many KPI initiatives lack: "framing".
The idea is simple: if you have to film weekly an episode, you need a team of writers- one is not enough.
But in order to ensure that, you need to have them all share a conceptual framework, so that any writer working on that series delivers something that can feel "part of the storyline".
Few business books, one from the 1970s, one from 1980s, and the last one from the 2020s:
_ 1978 Herman McDaniel "an introduction to Decision Logic Tables"
_ 1985 Bodily "Modern Decision Making - A Guide to Modeling with Decision Support Systems" 0-07-Y66152-9
_ 2020 Parmenter "Key Performance Indicators - Developing, Implementing, and Using Winning KPIs" 4th edition 9781119620792
The first book is interesting: went through it in Andersen's library in Turin while working from 1988 on Decision Support System models, and along with the Iterative development and product support side of company's methodology, used it to create... my own method to quickly develop DSS models.
Which, actually, were basically collection of equations fed into a tool that used linear regression within a multidimensional framework.
The second one has another funny side: I purchased it out of my own pocket (as did from 1988 to support working in multiple industries), but was useful for that method design- based on IFPS, it is still useful in terms of concepts, as it contains multiple case studies, and covers (at a macro level) most of the issues that I found times and again across the years whenever worked on KPIs, models, management reporting.
Incidentally: as discussed at the beginning of this article, the concept is that your should consider both the quantitative and the qualitative- using some creativity, when needed, to shift the latter to the former, notably in models that have to mix both.
Examples: vendor evaluation models, organizational design models used to decide the optimal staffing by mix of experience competence and other parameters, etc.
I used the concepts and models also while helping to design business and marketing planning for startups, e.g. to introduce some "sensitivity analysis" to see which factors could influence the implementation.
Were also useful to define, from late 1990s, how to allocate my time across different customers to optimize variety (to keep my knowledge-based network happy and skills alive) while balancing revenue by risk, channel, line of activity.
Managing your own "business pipeline" actually, also if you are a multi-customer freelance that coopts others in specific missions (even in background, to cross the Ts and dot the Is) is actually made easier if, after having covered the "business backbone" covering costs and a minimal operational cashflow, you have a way to proactively consider each "tremendous opportunity" in terms of potential benefits but also potential risks.
Just using the "Genghis Khan" approach of "taking whatever" sometimes if feasible- but, in our data-centric times where being local is not enough, risks making yourself and your organization an indifferentiated commodity that, eventually, could be replaced by either cheaper resources "orchestrated" wisely, or even by cleverer AI-fuelled tools.
The last one instead is the 4th edition of a book that purchased after returning in Italy in 2012, to update my knowledge, and that found useful also to compare with my own prior experience; it comes with a toolkit (files, templates, etc) that might be useful.
Again, as I did in the 1980s, purchased it out of my pocket along with books on WCM (World Class Manufacturing) and other business books (e.g. the IFRS "annual" few years back) to update my skills.
And, as it happens often...
...eventually all that reading (and more) was useful in my missions in Italy since 2012.
As for non-business books...
... I think that movies would be a quicker way to think across few lines useful while defining or using KPIs and the associated organizational paraphernalia.
About measuring and delivering:
_ thank you for smoking https://www.imdb.com/title/tt0427944/
_ oppenheimer the real story https://www.imdb.com/title/tt27192512/
_ the pentagon wars https://www.imdb.com/title/tt0144550
About processes can fail or require fixing while ongoing
_ charlie wilson's war https://www.imdb.com/title/tt0472062
_ black hawk down https://www.imdb.com/title/tt0265086
_ by dawn's early light https://www.imdb.com/title/tt0099197
About how signals (and KPIs) to be interpreted correctly require "local" knowledge (and willingness to accept the results):
_ seven days in may https://www.imdb.com/title/tt0058576
_ rogue trader https://www.imdb.com/title/tt0131566
_ the big short https://www.imdb.com/title/tt1596363
_ margin call https://www.imdb.com/title/tt1615147
_ too big to fail https://www.imdb.com/title/tt1742683
And I would like to close by sharing my comment to a funny meme that a Mensa friend posted this morning- that opens the door to something that can sink your own KPIs initiative into a powerful engine for organizational attrition.
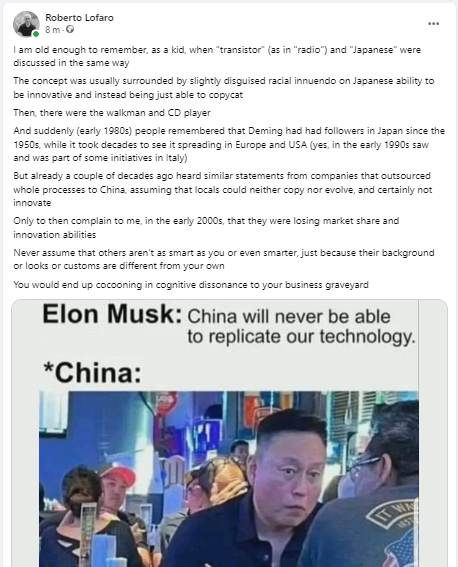
The rationale? Why replicate technology, when you have already brains able to develop something similar yet different (look at the visual, and you will see the funny side).
Anyway, this theme "they will not be able to" is something that has been with us since centuries, e.g. remember that it was well into the XX century that universal right to vote was not linked to census or sex or educational titles.
Ditto access to professions.
And even in the late 1990s to early 2000s, foreign non-white friends told me their stories of discrimination, and I remember meeting a Sikh with his traditional headgear in Bruxelles telling me, probably after a job interview where the focus was on his headgear and not his qualifications or experience, how lucky I was to look how I looked (white, christian).
A final critical point to keep in your mind: you do not need to be overtly restrictive, but by designing KPIs you might be incidentally exclusive, and behave as those Machine Learning models that "learn from the past" to propose the future.
Yes, a past based on racial, sexual, census bias projected into the future.
And not on a society that, by the definitions of "data centric" and "contextualization" that I gave above, still has to get used to the concept that "continuous learning" is not a matter of turning training into a frantic collection of "promotion points" as if it were all a game, but implies creating fertile ground for further learning and integration with experience.
In a data-centric society, "continuous learning" is both individual and organizational, and a decent KPIs design and evolution approach can actually help to adapt without losing sight of your organizational purposes, and integrate (not just extract) value from all those with the potential to contribute.
Only: beware of those offering off-the-shelf designs that "surf" quickly over the boring assessment and contextualization, and even offer to do those boring part as a quick, one-off set of activities.
Keeping your organizational culture and KPIs aligned has always been a Sysyphean task ADD LINK, but, as it was for the shift from pre-nuke policy to post-nuke, the decision-time cycles are shorter and shorter.
While some operational processes will be accelerated but not that much (e.g. bread still needs a certain amount of time), all the signs are there for organizational evolution to be in the future even more a matter of listening and continuous integration than policy setting barrelled down from the top.
As I said decades ago also to customers and partner- change is a constant, while many change initiatives (including performance, KPI, EPM, etc) live it as a crisis solved by yet another set of organizational tools (training, coaching, software, measurement) that attempts to bring about a "steady state".
Instead, the concept within the post-COVID Recovery and Resilience Facility was, in my view and experience on change within organizations, a step in the right direction, i.e. ensuring continuity while also reinforcing the basic building blocks to ease future change, even pre-emptive change.
The devil is within details- and certainly the National Recovery and Resilience plans (not just the Italian PNRR) had many details and "zombies" resurrected from drawer and political earmarks.
Resilience per se anyway should not be considered the new mantra, just a tool.
Otherwise, would become yet another implementation of a variant of the "military-industrial complex" discussed by President Eisenhower in his farewell address, i.e. a bureaucracy that, as with any bureaucracies, keeps finding new ways to justify its own existence and, potentially, expansion.
As in the movie "The Blob" https://www.imdb.com/title/tt0051418
I shared in my books since 2012 (and my e-zine on change 2003-2005) various cases and discussions on the theme.
You can see the outline of each on this website, and you can also search across the content of all the books; each book page contains also download links.
If you want to download massively, you can just visit my Leanpub author's page, where you can see the tag cloud of each book, and then download for free (just set the price to zero) or buy the digital version (in 2024 will revise, update, and republish most of them).
If you are interested in the paper version, just look at the ISBN within each book, and search on your favorite Amazon website.
See you at the next article.