Viewed 11426 times | words: 3446
Published on 2020-01-30 22:28:00 | words: 3446
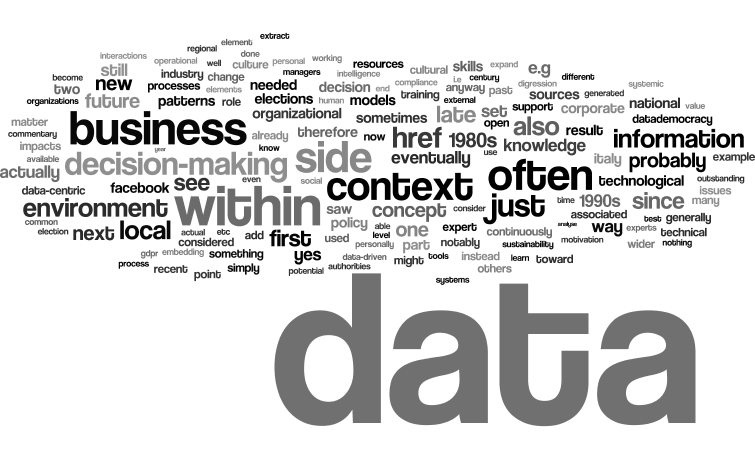
Yes, future, as when it comes to decision-making, way too many organizations still integrate external data as if we were in the 1970s, not entering the 2020s.
As some of my frequent readers probably noticed, since January 1st I changed my publishing approach.
First and foremost, I shifted on Facebook and Linkedin commentary on news, respectively general/politics and business.
Then... well, have a look at the About page.
Instead, tonight I would like to share again, and summarize plus expand (no, it isn't a contradiction) some ideas.
About what? Cultural, organizational, technological change initiatives within a data-centric society.
As I wrote often in recent articles, a data-centric society implies delegating across a wider spectrum of actors roles that traditionally were limited to few experts.
It is anyway nothing really new: even something as ordinary as writing was a "technical" skill.
From data, to big data, to data flood
The amount of data will just increase- and data exchanges between State, local authorities, business users, and individual citizens (and any association thereof) will out of necessity evolve and become, if not continuous, more frequent.
It is not just a matter of quantity- yes, we will continuously generate and consume data.
But it is a matter of quality- associating data and data generation with contextual interaction of data.
It is not just because you have data that you have to use them- already in the 1990s I was talking, while presenting business intelligence and knowledge dissemination solutions, of InfoGlut- Information Gluttony.
Or: taking on more data than you can possibly "absorb".
It was the beginning of the Internet open to commercial uses in Europe, but we were already seeing more data than we had generally organizational and technological capabilities to process.
Example: for some projects within the retail industry, in the 1990s it was common to hear that when processing details, they balanced volume vs. timeframe, e.g. having access to more details instanteneously, and reducing the level of detail as the time horizon extended.
Still, the data back then were generally produced by "certified sources".
With smartphones, sensors everywhere, etc. we have a variable amount of potential data sources providing information either continuously, or with a schedule that isn't predefined.
Most corporate information systems right now simply ignore those data, others seem focused on eventually collecting everything.
But, in the end, it is a matter of decision-making processes.
Decision-making and decision-makers
On the purely IT side, since the late 1980s my routine knowledge updates have been mainly "data centric", with a focus on data collection, structuring, dissemination, representation- to support the other side.
The interesting part is that, while the impacts of the "human side" is long-term, their value is often underestimated- probably also because humans up to a point are more flexible than systems.
And, often, it was the "human side" that ensured that the "technical side" delivered the desired outcomes within the context.
As usual, I consider "technical" any "focused", specialist skill: be it a computer programmer (by department: IT), an expert in risk models (finance), or an expert in designing training curricula or skills evaluation models (human resources) are all "technical", in my view.
Of course, demand is higher on what is "trendier", and usually the "technical-technological" (IT) side has a higher ability at connecting with e.g. ongoing compliance trends, notably to justify the expenditure and the associated "Return on Investment".
Since the late 1990s, more often than not also supposedly purely IT projects had a profile closer to those that I saw in the late 1980s while working on Decision Support Systems: being cross-domain, the cultural and organizational elements had major impacts.
I agree with those who say that probably CIOs will eventually disappear: there is a technological element that requires a "technological oversight and consistency" role, akin to the CTO, but the "information" side of the "Chief Information Officer" should become embedded within any CxO role.
Eventually, most managers and senior managers will have number-crunching skills that just a decade or two ago were associated with "rocket scientists".
Personally, since late 2017 used SAP training courses and presentations on architecture and cross-domain processes as a way to see how Industry 4.0, Business 4.0, and overall "digital transformation" could become "embedded within any CxO role".
Or: no data, no business- and this will include both "quantitative" and "qualitative" data collection and processing.
Therefore, the skills and abilities that will be needed in the future will expand- covering both the "data-centric" and the ability to see and analyse the "wider picture".
Recently Facebook reminded me of an old post of mine (2014): "if you want to learn what motivates others, you need to watch them in their own environment, not just write about them on Facebook", and I received a comment: "Are you an expert on what motivates others?".
My answer: "If you work on cultural and organizational change, you learn that".
My interest started as a hobby (cultural anthropology and archeology, as well as comparing Constitutions to see how this was mirrored in cultures), and then had a chance to "test ideas" as a teenager, before entering business.
Actually, I was lucky in my career- as first in politics, then in sales (both as a teenager), then in the Army, I was able to study the motivational patterns of both individuals and groups or sub-groups.
And, in my first official job, for the Italian branch of a multinational, between 1986 and 1990, was first able to be on the receiving end of a "rating form" that tried to deliver motivation through some means that would imply an homogeneous set of opportunities and ambitions.
Result? If the shift was toward the monetary result, it created inflationary expectations: if your "outstanding" rating gives you, say, a 5% salary raise one year, but the next year "outstanding" delivers less, you can hear what I heard from some.
Moreover, after being sometimes told that it was a Gaussian distribution, and therefore there could not be too many "outstanding".
From 1988, in my role on decision support system models, discussed with people on the decision-making side the actual underlying logic.
I found curious to see similar models spreading around decades later, and repeating the same issues, almost verbatim- zilch social learning.
In the future, probably instead interactions across organizational boundaries will share not just data, but also their context and past impacts (a data-centric version of "lessons learned", embedding both quant and non-quant side).
Yes, there are many who actually sit at a table and redesign cultures, but, frankly, social engineering is often done in such a way to remove reality from the context.
Then, many are puzzlingly surprised when results aren't those expected.
As I wrote often in the past, the wider the impact of the change that you are focusing on, the deeper the commitment of those involved generally has to be.
As an example, also when working as a negotiator or teaching how to carry out interviews for business analysis, I discussed the context and aims: if the purpose, as it was initially in my case, was to "extract" the decision-making process as carried out, it was better to go where the decision-maker was in her/his own environment, so that you could actually see also what was done but not documented.
And I saw the side-effect of interviews for the same purpose done in a "neutral environment": over-rationalization, resulting in models that often were not delivering what was expected.
Litmus test? Currently we talk a lot about "machine learning", and splitting data into a "train" set, and a "verification" set, i.e data used to train the software, and data to see the actual performance on new data.
In my decision support system times, I was the "machine" who had to learn, and simply the first set of data was the one that along with the decision-maker applied the model to.
Then, often, on new data we went in parallel: to see if the choice by the one who understood business and what would be the impact of the new data matched what the model forecast.
In the end, the point is always the same: I disagree with "fixed patterns", my experience say that having a "catalogue of patterns" is more appropriate- and it is the context that should define which one should be used.
Anyway, the context isn't just a yes/no choice, is a matter of sensibility, of identifying what in other domains are called "hidden variables".
Before talking again about the future, I will do a small digression.
Digression: regional elections played under a national rulebook
I am from Italy, and therefore in this case I am referring to the recent regional elections, notably those in Emilia-Romagna.
My commentary to the elections is on Facebook, and you can have a look at the voters' flows as well.
The key point are two results: the centre-left ("red") retained control of the regional government, and the centre-right ("blue/green"), while losing, did so with a wide gap, but with a result strong enough to increase its elected representatives, and basically turn a traditionally "red" region into a contestable one.
But what surprised me was that the centre-right "played" by a rulebook that seemed tailored for the national elections.
Ruffling local feathers on some issues could have been useful in a national election, where the local element (and ruffled feathers) would affect only local vote, in a region that was anyway considered "lost".
But in Italy, a country of almost 8,000 towns and villages who routinely remind of past quarrels between them?
Asking for the local vote while insulting the locals with generalizations and with some antics that were out-of-the-line with the local context was quixotic.
But, probably, it was a play to position for the next elections, i.e. a polarization move, or: sacrifice a local election but play it to show as a personal success and to build up a territorial base for the next round while confirming your own positioning (yes, I know that some friends will recognize some "patterns").
A gamble.
Meanwhile, anyway, this scared allies more than competitors, as probably they do not know if, in the next election, they will be "sacrifice toward the next step" or "allies toward a common goal".
This digression has actually two purposes.
Choices have impacts, but sometimes those providing information might have a different agenda, and this could therefore distort the context (or perception thereof).
The context you assume if you consider that there is a shared motivation, and the actual context if you consider the temporary joint motivation vs. the final target.
Data and context
In the 1980s and partially 1990s, most of the data considered were generated within the company, e.g. I remember that also adding macroeconomic elements was restricted to few types of reports for few customers.
Gradually, more external data became run-of-the-mill, integrated within management reporting, dashboards, etc- but still a fraction of what was available (except in few cases when compulsory due to regulations, compliance, etc).
On the "tools" side, in the 1990s became common for any manager to be able to use Excel (and, before that, Lotus 1-2-3), directly or through an assistant, to routinely "crunch" data way beyond what was available decades before, e.g. to have a report when needed, without involving IT staff.
Personally, I worked between the late 1980s and mid-2000s with more than half a dozen companies selling software solutions to enable the next step, i.e. "business intelligence", data warehousing, and the like (and the staarting point often was "industrializing" some solution derived from overgrown and unmanageable Lotus 1-2-3 or Excel spreadsheets).
Sometimes I was on the "technological" side, sometimes I was on the business side, most often connecting the two.
In the 2020s the sheer volume of data and number of potential interactions between evolving compliance, data, and business processes (and relationships) will turn data into something too important to be left just to data experts (yes, I am paraphrasing Von Clausewitz).
Moreover, within this decade I am relatively confident, from recent "tests" I had to do just by chance in Italy since 2012, but with reference to shared regulatory frameworks in EU, that eventually our "regulation by industry" will have to be converted into something else, more "systemic" and continuously adapting, probably by continuously "polling" consensus from all the stakeholders.
My personal complaint in Italy is that often I see that our business culture is still pre-industrial, as if we were still passing operational knowledge from father to son, while instead living in the XXI century.
On the regulatory side, I often use the concept of "frankenlaws", as in Italy we import regulations and associated constraints without evolving our culture, and often simply "layering" new formalities on top of the "informal"/"formal" pre-existing national culture.
Net result: one rule / regulation, multiple implementation variants, even within the same supposedly regulated industry (and this in multiple industries, in my limited and recent experience- so, I do not know how widespread it is).
Personally, since mid-2018 expanded within my publications the data-driven element, e.g. with the book and series of articles that you can read at the GDPR page.
Part of my knowledge update included also expanding my knowledge on operational frameworks that result from the shift from Corporate Social Responsability to Sustainability, notably sustainability at the systemic level.
Then, during the summer 2018, started adding also elements of what is now part of DataDemocracy.
The concept is simple: until now, most of the data that I saw used in decision-making and management reporting since the late 1980s were produced from within business organizations, but the push from the late 1990s for e-government resulted eventually in the continuous release of OpenData.
But, so far, most of those data are simply "dumped into a public lake", as the public authorities producing them lack often the resources and skills needed to analyse and derive behavioral change initiatives (a.k.a. "policy") from them.
Our current data-driven reality evolves too fast for the reaction times and agenda-setting processes designed for centralized states in the XIX century, and barely updated in the XX century.
Actually, I should amend on that: often, they have the resources and skills needed to carry out what are their "main purposes", but nothing more, and nothing less.
Therefore, once the data are available, integrating them within corporate decision-making might actually extract value.
Training the next generation of decision-makers
I hail from a country where 95% of the companies are so small that they focus on doing what is needed today, and within their organization they lack the resources, organization, culture, and demand to justify (and maintain) even a single full-time data scientist.
I posted few days ago on Facebook an image and commentary sharing some data from national statistics, if you are curious.
There are at least two upsides of starting now to integrate open data from State, local, and supranational authorities within line managers' decision toolset.
First, it will expand their concept of "data" to the wider context: sustainability and systemic thinking aren't just an add-on to existing practices, both require a redesign of mindsets (and training patterns), and a degree of flexibility.
Second, while being "open" these data sources are still "professional", but with different motivations and no possibility to directly influence, time, content, structure, preparing for the future type of data-driven interactions.
Instead, since the 1980s I saw that generally the data exchanges between different organizations (e.g. the first EDIs that I saw in the 1980s) were based on a kind of "hub and spoke" concept (or driver/driven paradigm, to be polite).
So, open data could be considered a "controlled environment" to test new decision-making approaches within a "fail safe" environment.
This should help both business and IT to conceptually prepare to have and treat "self-service" data enter the corporate environment and influence decision-making, and define the associated "policy framework".
Alternative? Building a 1970s-1980s "data firewall" that gets data only when have been already pre-digested and ingested within an internal, corporate environment.
A recipe for being late on extracting value from data- not necessarily generated internally.
It is akin to the difference between retaining the gold, and retaining also all the debris that surrounded the gold when it was extracted.
At the beginning, the concept could just to limit the number of sources that are considered "acceptable"- a kind of "avoid fake data" policy, by certifying the data quality of sources.
As an example, you could accept data from Eurostat, and refuse similar data from a local authority, knowing that this will add a delay.
Or: introduce a policy to accept real-time data from certain "sensor data providers" whose working rules are compatible with your own standards.
I talked above of "policy framework", something closer to the concept of the GDPR "evolutionary part" (privacy by design, privacy by default), more than simple "guidelines".
Riding the data flood wave
Building a "data dam" isn't going to be worth a damn, business-wise.
To extract a competitive advantage from the future data environment, notably within a "smart city" environment where everything and everybody will be both a data producer and data consumer, you will need to have potential "consumers" of data to be somewhat more proactive, more "data literate".
Literate in terms of tools, but first and foremost expert on the business side, not on the data tools side.
Then, they can trasfer to experts the "industrialization" of specific data investigations that might be turned into a routine process.
At the same time, "embedding" external information into their decision-making should have a way to make transparent toward others the constraints and reliability of the information, to avoid past issues that sometimes generated issues in data warehousing and business intelligence.
As an example, data that made sense at the operational level, when aggregated, might show a trend that isn't there, if the relative context of each set of data isn't properly "weighted".
It seems intuitive- and if you think at few items, it is.
But when you add dozens of points at the bottom, and consolidate through three or four levels, it is already more complex.
If you add thousands of points, each one should have a way to convey its own context along with the data, so that, before embedding those data within decision-making, the information itself is "contextualized".
To close on a personal note: yes, as an experiment, as part of my knowledge update over the last few years I have been "testing the ground" on myself (eventually indirectly producing a benefit for some customers by recycling old and new concepts, when it was useful).
And will add more material within the subsite that includes the DataDemocracy concept, the BookSite.